Decoding Nvidia's vRAN economics
Nvidia has sketched out a vision for AI-enhanced vRAN, but there are questions about how well-suited power-hungry GPUs are to the task.
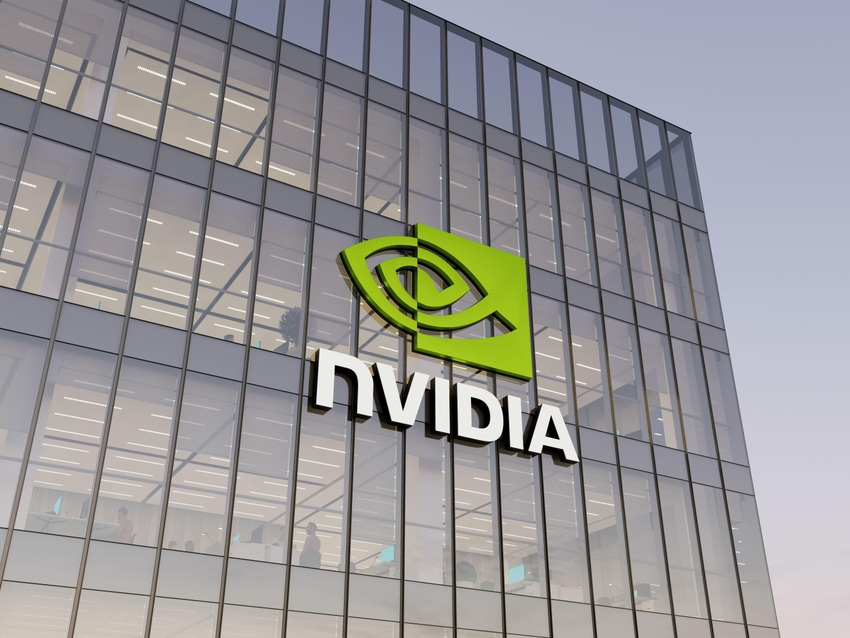
Nvidia — the hottest tech company on the planet — has a vRAN play.
The idea is that Nvidia GPUs are well-suited to the parallel processing tasks in baseband software and outperform other general-purpose processors in vRAN applications. In addition, says the company, GPUs offer better economics than dedicated RAN silicon because they are programmable and produced in large volumes.
This is, in essence, a standard market positioning pitch. No doubt, some of it is correct, and some is open to interrogation. Nvidia is an incredibly successful company, so as telecom industry analysts, we take its vRAN pitch very seriously.
Nvidia's claims of superior RAN economics are a good place to start. A workshop on "vRAN Next: Driving Innovation in Wireless Towards 6G" at Nvidia's recent GTC AI Conference and Expo worked through the basic business case for using servers built around its Grace Hopper Superchip for vRAN.
Guest speaker Ryuji Wakikawa, vice president of the Research Institute of Advanced Technology at Softbank (a Japanese operator and longtime Nvidia vRAN development partner), set out the logic. According to Wakikawa, a typical 5G distributed RAN operates at around 30% efficiency based on the average amount of physical resource blocks (PRBs) consumed to serve users. This arrangement works fine in the sense that it gives headroom for traffic growth and allows sites to serve peak load conditions. It is, however, fundamentally inefficient.
The Grace Hopper Superchip does not change this efficiency problem on a like-for-like basis. For a typical distributed RAN cell site, it is over-specified and expensive. Thus, the proposal is to move to a centralized RAN (C-RAN) deployment architecture that aggregates baseband from multiple sites onto a cloud infrastructure built using Grace Hopper servers.
C-RAN – its time will come… someday, maybe
The basic math is as follows: a single Grace Hopper server (we assume a single-slot server) can support 20 x 100MHz cells, and a standard rack can support 20 servers, which equates to 400 cells per rack. The GPU usage to serve 400 cells (calculated from the PRB usage) would consume "something like 60% to 70%" of the capacity of the rack, according to Softbank's Wakikawa. In other words, greater efficiency is possible by multiplexing the RAN workload.
"People say Grace Hopper is so expensive, but if we serve 20 cells, the cost is divided by 20 and it's going to be very competitive," Wakikawa says. "It's cheaper than the existing base stations available today."
This is an interesting analysis. The idea that C-RAN can make more efficient use of compute and lead to lower cell site costs has been around for decades — I wrote "Time to Check In at the Base Station Hotel" 19 years ago, for example. Nvidia has adopted the idea but has not, so far, changed the economics of C-RAN.
Classically, the C-RAN business case does not fall on server utilization metrics. Instead, it falls on the need for fronthaul transport (which in turn consumes power, requires investment, etc.) and related issues such as resiliency, failover and the impact on operations.
The challenges of centralization can be overcome — Rakuten Mobile (also in Japan) is built this way — and surely C-RAN's time will come someday. See my December 2023 article, "Analyzing cloud native RAN topologies," for more.
Right now, though, C-RAN is deployed only sparingly. And not everyone believes a C-RAN hub with an all-seeing "super brain" to control radio resources across the service area is the way to go. An alternative view, for example, is to integrate more capability into high volume, low cost radios and centralize control and management at Layer 2/3 and above.
Inference at the telco edge
The next stage of the Nvidia argument is to use vRAN as the anchor tenant for multipurpose edge cloud infrastructure. The idea is that the telco can resell surplus GPU resources to AI service providers. "If we can serve AI inference at our base stations, there's a lot of opportunity," says Softbank vice president Wakikawa. "We will transform our business from telco centric to more AI centric."
This is an interesting idea but, again, not wholly new. In fact, the industry has spent the last five years discussing edge cloud infrastructure. The difference, perhaps, is that because Nvidia is involved, we are now talking about AI-driven edge services (a.k.a. inference). Network access is essential to future AI services, runs the argument, and services hosted at the telco network edge will offer superior performance. This logic of "inference close to the user" probably best explains Nvidia's interest in the RAN market.
Where C-RAN is deployed today, RAN functions are deployed on dedicated servers/racks optimized for RAN. Heavy Reading is not aware of wide-area vRAN being deployed as a workload on a multi-tenant cloud infrastructure. Moreover, operators will be cautious about adopting a multi-tenant model due to reliability, resiliency, security, regulation, etc. There are potentially ways around these challenges as cloud RAN evolves, but the timescales are hard to judge. How about 2028 as a guestimate for commercial deployment of wide-area public network vRAN on multi-tenant infrastructure?
Toward AI-native RAN
From my independent analyst perspective, it is fantastic to see Nvidia investing in the RAN and bringing its R&D culture to mobile access. And credit to the Japanese operator Softbank for starting the hard preparatory work needed for commercial deployment.
On the economics of general-purpose compute for RAN, Nvidia has sketched out a reasonable vision — enough to get us interested. But there are questions about how suited expensive and power-hungry GPUs are to vRAN that the company has not quite answered yet.
Keep in mind, also, that this article only covers half the story. It gets more interesting with the emergence of "AI RAN." Imagine every cell is a neural receiver perfectly tuned to the environment, load conditions and user requirements, and then map that capability to AI-driven end-user services — all running on AI-enabled infrastructure deployed at the telco edge.
This triumvirate of developing AI RAN, running on AI infrastructure and delivering AI services is more compelling and ambitious than a business case built around optimizing existing RAN architectures to suit the needs of general-purpose silicon. And it is a great subject for another blog.
About the Author(s)
You May Also Like